Build-to-rent (BTR) buildings represent a fast growing trend in the real estate sector, characterised by properties specifically constructed for long-term rental rather than sale. These developments cater to a growing demographic of renters, including young professionals, families, and downsizing retirees, who prioritise convenience, flexibility, and a professionally managed building.
The use of AI to determine lease prices in BTR buildings represents a significant innovation in the real estate industry. This optimises rental pricing by analysing a multitude of factors such as market trends, local economic conditions, property amenities, and tenant demographics and leasing history. This system ensures that lease prices are competitive and fair, dynamically adjusting to real-time changes in supply and demand, adapting to the tenant’s willingness to pay.
The Challenge
The primary objective of all BTR operators is to reach full occupancy at the highest achievable rents, as quickly as possible.The potential risk to under or over price is high, leading to costly voids and delays.
Determining lease prices for BTR properties using existing spreadsheet based methods presents several significant challenges. Pricing is done at an individual unit level (but spread over an entire building level) rather than truly understanding the tenant and unit particulars. The traditional approach is highly manual, requiring extensive data collection and analysis, which is both time-consuming and prone to human error. Property managers must constantly monitor market trends, local economic indicators, and competitive pricing, often leading to frequent back-and-forth adjustments.
Furthermore pricing is currently based off a narrow set of market properties such as market competition and building specifics (e.g., proximity to elevator, floor, presence of a balcony etc.) rather than tapping into tenant demographics (e.g., job, family / marital status / income / duration of tenure) and tenant history (whether they have negotiated a lease, their earnings, time of lease or lodged a complaint). This manual process can result in delayed reactions to market changes, causing either overpricing that leads to voids or underpricing, reducing potential revenue.
Additionally, the lack of real-time data analysis means that pricing decisions may not accurately reflect current market conditions, leading to inefficiencies and potential financial losses. Overall, existing approaches not only increase operational workload but also diminish the ability to optimise rental income and maintain competitiveness in the dynamic rental property market.
The Solution: Artefact’s suite of dynamic pricing strategy tools
Artefact has developed several advanced solutions for lease pricing in BTR applications, including customer segmentation, forecasting, and reinforcement learning. A phased approach can also be particularly effective, allowing property managers to start with simpler methods and gradually adopt more sophisticated techniques as data availability and system maturity increase. All suggested solutions build on traditional methods of pricing by tapping into lessee specific factors including their willingness to pay, demographics and tenant history.
By considering a multitude of variables such as location, amenities, historical occupancy rates, and tenant demographics, AI-driven pricing models offer precision and adaptability far beyond traditional methods. Primarily, these advanced strategies:
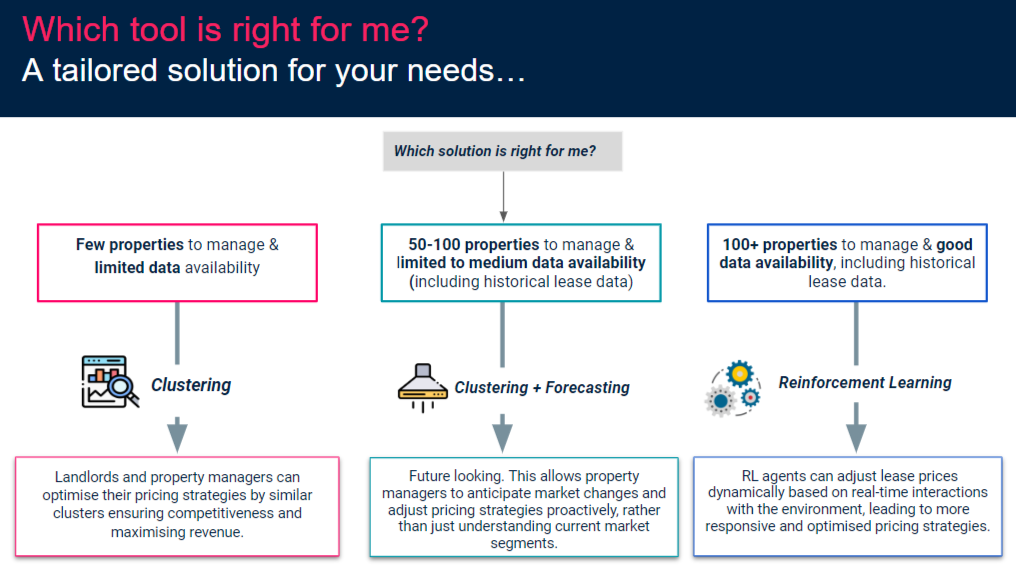
Solution 1: Pricing driven by customer segmentation
Segmentation can be a powerful tool to support lease pricing. By grouping units with similar characteristics (for e.g. size, amenities, and location within the development), as well as grouping similar customers based on their incomes and demographic characteristics you can start to identify patterns within groups and adapt the pricing accordingly. This approach provides a much richer view of the rental market, allowing agents to set profitable yet competitive rent prices, rather than a “one size fits all strategy”.
Segment based pricing is easy to implement and understand for the end users. However, the effectiveness of clustering relies heavily on the quality and completeness of the data. Inaccurate, outdated, or incomplete data can lead to poorly formed clusters, resulting in suboptimal pricing strategies.
Solution 2: Smart Demand Forecasting
While customer segmentation helps us understand current market trends for lease pricing, forecasting demand takes things a step further. By making use of large existing registers of historical lease data, economic indicators, and future development plans for the area, we can reliably predict how demand for a particular property can change over time and consequently how pricing can be optimised to meet demand. Key factors to include in the analysis are:
Similar to segmentation, effectiveness of forecasting relies heavily on the quality and completeness of the historical lease registers available. Reliable insights will require continuous monitoring and fine-tuning by skilled professionals to ensure they remain effective and relevant in changing market conditions.
Solution 3: BTR Dynamic Lease Pricing Asset
Reinforcement learning (RL) systems can be highly effective for implementing dynamic pricing strategies BTR properties, particularly in situations where there are a lot of apartments to manage. These systems can be designed to operate within predefined pricing bands, ensuring that lease prices remain competitive while preventing drastic fluctuations that might deter potential renters. By continuously analysing real-time data on market trends, tenant behaviour, and occupancy rates, RL algorithms adjust prices within these bands to optimise revenue and maintain high occupancy levels.
In practice, an RL agent would be deployed as described below:
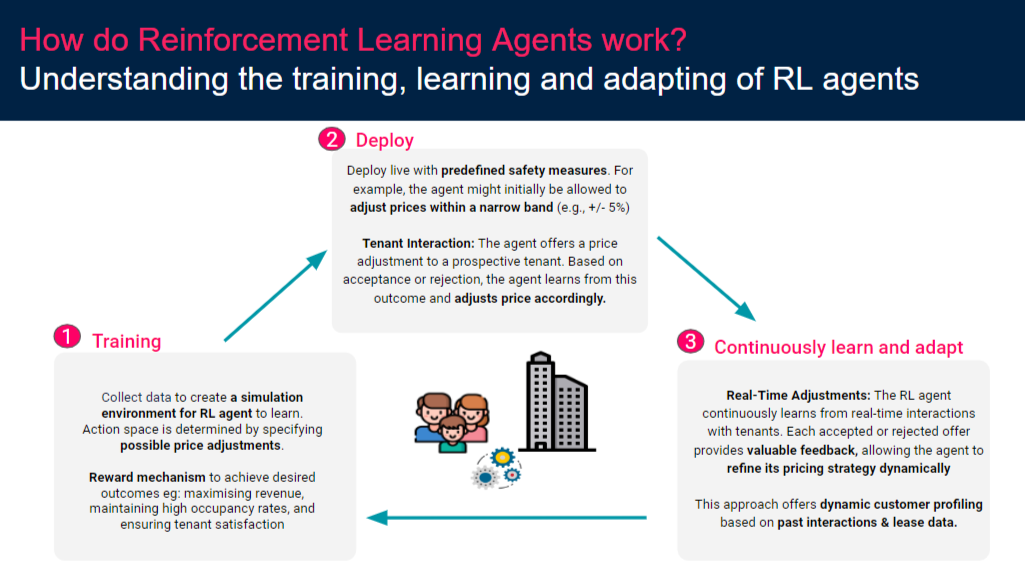
Using RL to set lease prices for properties introduces several complexities and risks including the need for vast amounts of high quality data in order for the agent to learn and adapt continuously. To mitigate the risk of drastic price fluctuations as the agent learns in the live environment, lease price adjustments should be kept within a narrow band to avoid tenant dissatisfaction and market instability. In addition, ensuring that human presence is maintained in the loop supports the fair and robust outcomes in the pricing strategy. Periodic retraining should be conducted to incorporate new data and adapt to changing market conditions.
While clustering and forecasting are useful methods for lease pricing, providing valuable insights into market trends and tenant segmentation, they often fall short in addressing individual tenant preferences. RL agents, however, excel in this area by dynamically calibrating the Price Elasticity of Demand for each tenant. This allows agents to offer personalised pricing strategies that more accurately reflect individual tenant behaviours and preferences, leading to more effective and tailored leasing solutions.
As the BTR sector continues to grow, the integration of AI in pricing strategies promises to streamline operations, reduce vacancies, and contribute to a more efficient and responsive rental market.
Why Artefact?
Artefact is a leading global data consulting company dedicated to accelerating the adoption of data and AI to positively impact people and organisations. We specialise in data transformation and data marketing to drive tangible business results across the entire enterprise value chain. Artefact offers the most comprehensive set of data-driven solutions, built on deep data science and cutting-edge AI technologies, delivering AI projects at scale across the property sector in the UK.
We are trusted partners to property businesses across residential, commercial, industrial and specialist asset classes. Our partners include FTSE 350 listed companies and similar-sized private organisations. With over 20 years of experience in real estate, our dedicated property team includes experts and chartered professionals in property valuations, urban planning, development, and financing.
Our previous work ranges from developing data-led dynamic strategies with our clients – informing them where to play and how to win in their chosen markets – to major operational changes, such as establishing new business arms and propositions. We have worked in every stage of the property lifecycle, from land acquisition to ongoing maintenance, and have worked with clients to scientifically improve these processes.